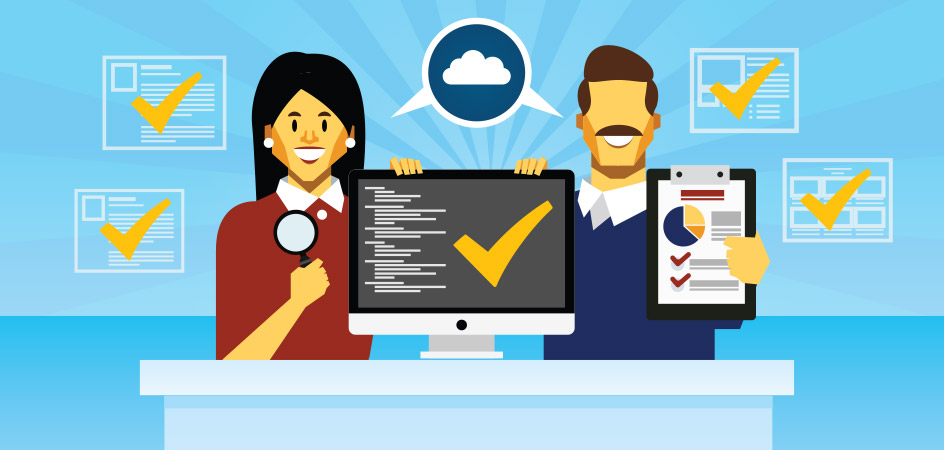
Setting Up Your Big Data Analytics Flow: Challenges and Solutions

Posted by Walid Abou-Halloun Date: Mar 16, 2018 9:54:33 AM
If you’re used to traditional data analysis, you may be wary of utilising any sort of big data analytics. It can be very intimidating, especially to those who have no idea on how to do it.
According to CIO Insight, three out of five people in leadership roles have stated that failure to get on board with big data could lead to obsolescence.
Certainly, there are challenges to using big data analytics. However, these can be overcome with the effective use of the big data lifecycle. Read on to discover just how you can maximise this approach.
What is Big Data Analytics?
As the name implies, big data analysis is a much larger scale of data processing.
Unlike its traditional counterpart, it involves different techniques and technologies, particularly hardware appliances, software tools, analytics tools, and graph-based data management systems.
What are the challenges involved with it?
One of the biggest problems surrounding big data analytics is its usability when compared to more traditional approaches to data collection.
Though you may have invested a lot of time and resources in developing a way to analyse and report data, you can still end up dissatisfied with how this data has been organised. How, you might ask?
For example, a data scientist may be looking for specific information, however, it has been filtered out due to the IT practitioner’s objective to have rapid report generation and fast responses.
Meaning, although data warehouses are excellent for putting data onto spreadsheets, they are quite limiting when it comes to discovering data. This creates demands from analysts who want access to the raw data instead of just funneled requests.
This goes to show the tension between the traditional data warehouse and the now rising demand for new analytical tools that enable more queries from a greater number of users than ever before.
As an organisation, you need to find a way to discover whether these new technologies are valuable or not, and if they can be included in your information management structure and deployed effectively.
There are even more challenges that come with this, and we have listed them below.
1. Big Learning Curve
Although some forms of analytic techniques are simple to grasp, many of them do involve a steep learning curve. Sure, it is easy to download an open source software or a graph-based database system, but that is just the tip of the iceberg.
Developing applications that use these platforms can also be a very complex process, unless, of course, the developer is experienced with parallel code development and data distribution.
2. Changes in Data Lifecycle
Compared to the traditional process, the data lifecycle demands big data analytics in more ways than one. While traditional data warehouses are more often than not just populated with static data sets, live data can be streamed directly into a big data analytical application for real-time integration.
3. Existing Infrastructure
Due to many years of investment in traditional data warehouses and frameworks, certain approaches to data management have already been set in stone.
How does this become a challenge? Simple. When you become so used to a certain way of extracting data, it can be hard to switch over to a newer approach—even when most of the time, a more efficient approach is available.
4. Intent with Data
When it comes to data instances, processes are created for a specific purpose. The challenge lies here: as big data applications seek to repurpose data for analysis, the original intent of the data can differ quite drastically.
This then implies a need for greater quality, data control, and consistency.
5. Size and Duration
Who wouldn’t want access to massive datasets? There are direct implications, however, when dealing with the purer aspects of data management.
In fact, the desire to retain these large datasets (for potentially coming up with new analyses in the future) clashes with the transitory characteristics and rapid turnaround of numerous data streams.
As a result, enterprises are forced to make capital and investment acquisition decisions to support data retention.
What can be done to solve these challenges?
Statistics show that 85% of companies are trying to be data-driven. Yet, only 37% of them have been successful—and big data has played an important role in it.
To address the challenges and sheer volume of big data, this step-by-step methodology can help you organise any and all activities and tasks in acquiring, processing, and analysing data.
1. Business Case Evaluation
The lifecycle of a big data analytics starts with a well-thought-out business case which identifies the goals of carrying out the analysis.
After careful assessment and approval, the business case will be used during hands-on analysis. This evaluation process helps decision-makers understand which challenges are to be encountered and what business resources shall be utilised. Identifying KPIs during this stage can aid in formulating the criteria and guidelines for evaluation.
2. Data Identification
Data identification is all about pinpointing the datasets needed for the analysis project and, well, their sources.
By identifying a wider variety of data sources, you may be able to increase the chances of finding any hidden correlations and patterns. This is especially so when what is being looked for is unidentified yet.
The required datasets and their sources can be external or internal to the enterprise, depending on the nature of the business problems and the scope of the big data analytics project.
3. Data Acquisition
At this point in the cycle, data is gathered from all of the sources that were identified in the previous stage. This data will then be subjected to automated filtering, which removes any corrupt or irrelevant components.
Note that data may come in different forms—it can be a collection of files like data purchased from a third-party provider, or information that requires API integration such as data from Twitter. It varies based on the type of data source used.
Poor data can cost businesses dramatically, with some losing around 20% to 35% of their operating revenue because of it. Needless to say, it is important that data acquisition is included and closely monitored as part of the big data analytics lifecycle.
4. Data Extraction
Now, some of the data that has been identified as input for analysis may turn incompatible with the big data solution. How do you fix this? This is where data extraction comes in.
Dedicated to extract data and transform it into a usable format, this process is vital for data analysis.
The full extent of this extraction lies heavily on the capabilities of the big data solution and the type of analytics used. For example, extracting required fields from delimited textual data may no longer be required, especially if the big data solution can already process it directly.
5. Data Validation
Invalid data can skew your analysis results. While data structure can be pre-defined and pre-validated with traditional means, big data input can be unstructured and unverified.
What makes it even more difficult is the overall complexity of big data, which is why data validation focuses on establishing these complex authentication rules, and finally taking that stubborn (read: invalid) data out of the picture.
6. Data Aggregation
In some cases, data may spread out across multiple datasets. This means that these datasets will be required to join together via common fields, such as date.
In other cases, the same data fields may even appear in multiple datasets. No matter how the datasets are joined, a method of data reconciliation is required.
This is where the data aggregation stage comes into play. This stage focuses on integrating several datasets together to arrive at a unified view.
7. Data Analysis
From simply querying a dataset and computing an aggregation for comparison, to combining intricate statistical techniques and data mining to discover patterns or anomalies, this stage can be simple or complex depending on the type of analytic results required.
8. Data Visualisation
There is little value in being able to analyse massive amounts of data and finding useful insights if the only people who can interpret the results are analysts.
So, for this stage, using visualisation tools is the hero. Making the life of business users easier is definitely the way to go, and this can be done by presenting analysis results through graphic and visual aids.
9. Utilising Analysis Results
The final stage of the big data analytics lifecycle, utilisation of results is entirely dedicated to determining where and how the processed data can be further leveraged.
What are your next possible steps? How do you maximise the information acquired? These are only some of the directions you can take after going through all the stages of the methodology.
Big Data Analytics is Simple When You Have Experts by Your Side
If you’re still unsure of what to do when it comes to big data, don’t worry. Tapping an expert to help you set up and maintain a dynamic big data analytics flow is just a click away!
Contact us today to get started.